Far East Journal of Theoretical Statistics
International Journal of Statistics and Applied Mathematics
International Journal of Mathematics And its Applications (IJMAA)
International Journal of Mathematical Analysis
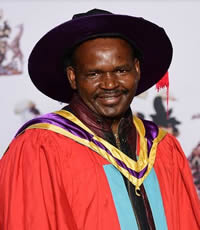
Student Bio
PhD in Mathematical Statistics of the University of Nairobi (2020)
Masters of Science in Statistics of the University of Nairobi (2006)
Bachelor of Education (Mathematics and Economics), Kenyatta University (1997)
Interested in research and data analysis using up to data software Director sdatacare and capacity builders Community service: Currently Pastor, Full Gospel Churches of Kenya.
Project Summary
Project Title : SYMMETRIC VARIANT TRUTH DETECTION MODEL IN SAMPLE SURVEYS - A RANDOMIZED RESPONSE APPROACH
Project Abstract
In every survey truthfulness is required so as to come up with valid data for decision making. Most surveys use direct questioning to collect data. This method does not yield reliable information when the topic under investigation is sensitive in nature. In such surveys, direct questions are not useful as the respondents will either refuse to answer the survey questions or, even if they do, may give false answers for fear of being known to have the sensitive characteristics. The less privacy a design offers, the more likely respondents cheat by disobeying the instructions thus giving very unreliable information which can lead to wrong decision making. In this study we have formulated a technique which we have called symmetric variant truth detection model. We have also formulated symmetric stratified truth detection model for analyzing stratified data. In this technique, we have used two randomization devises which do not required respondents to disclose their identity thus increasing their privacy leading to more honest response. After developing the models, they were validated by the use of data simulation as well as real life application. It was established that the symmetric truth detection models were more efficient compared to the asymmetric truth detection models. This study therefore recommended that researchers on sensitive information should use symmetric truth detection models as opposed to asymmetric truth detection models.