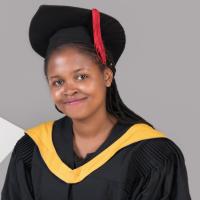
Student Bio
Shelmith Wanjiru Macharia: MSc. Biometry (UoN, 2020) and BSc. Statistics (first class honors, UoN, 2018). Shelmith is a recipient of DELTAS Africa - Sub-Saharan Africa Consortium for Advanced Biostatistics (SSACAB) MSc scholarship. For her thesis, she conducted a simulation study to compare the performance of Multiple Imputation Chained Equations (MICE) and K Nearest Neighbors (KNN) based imputation on missing Demographic Health Survey data under the guidance of Dr. Timothy Kamanu (UoN) and Mr. Paul Mwaniki (KEMRI Wellcome Trust Programme). Shelmith works as a data scientist at AJUA (formerly mSurvey). She is passionate about data science, machine learning and statistics. She is an R and Python enthusiast, and a speaker at R-Ladies Nairobi where she recently gave a talk on text mining in R. Shelmith volunteers as a judge at data science technical review bench, Moringa school and as a facilitator at various data science meet ups in Nairobi. Her hobbies are travelling and hiking.
Project Summary
Project Title:
Comparing the performance of Multiple Imputation Chained Equations (MICE) and K Nearest Neighbors (KNN) based imputation on missing Demographic Health Survey (DHS) data: A simulation study
Abstract
Background: Missing data is a common problem in Kenya Demographic Health Survey (KDHS) datasets. Complete case analysis is often used to deal with missingness which may lead to bias. Multiple imputation chained equations (MICE) allows for uncertainty in imputations and often uses linear models to impute missing values. Linear models may give biased estimates when a strict linear relationship does not exist between the response variable and covariates. Machine learning based imputation methods are an alternative to MICE since they do not require a linear relationship between the response variable and covariates but may underestimate the standard errors of estimates. This study seeks to compare parameters obtained from MICE and KNN based imputation as well as test whether KNN based imputation underestimates standard errors of estimates. Methods: Missing values in the KDHS 2014 dataset about children were substituted with medians and modes to obtain a complete dataset. Missingness was introduced to the complete dataset in different proportions of missingness. A hundred simulated datasets for each proportion of missingness were obtained. MICE and KNN based imputation were applied to each simulated dataset. Logistic regression models were fitted to the complete and imputed datasets. Differences between regression parameters from complete and imputed datasets were used to evaluate the performance of MICE and KNN based imputation. Results: KNN based imputation performed better than MICE. KNN based imputation underestimated standard errors in a number of cases. The proportion of missingness did not have an effect on the results obtained from imputed datasets. Conclusion: This study recommends KNN based imputation as a method that deserves further consideration in future.