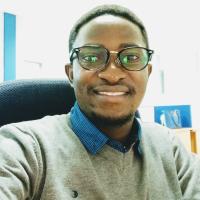
Student Bio
Abubaker holds a master's degree in bio-statistics from the University of Nairobi in Kenya and a first-class bachelor’s degree in statistics from Makerere University in Kampala, Uganda. His master's thesis was about the application of novel subsampling techniques for monitoring routine health indicators using the District Health Information System (DHIS2). This research was done in collaboration with the KEMRI Wellcome Trust Research Programme (KWTRP) in Nairobi with support from DELTAS Africa Initiative - SSACAB and The Initiative to Develop African Research Leaders (IDeAL). Abubaker believes that the training in advanced biostatistics methods he obtained from the University of Nairobi coupled with the mentorship in research that he got from KWTRP make him one of the best bio-statisticians in Africa. He hopes to apply his skills and knowledge towards improving health systems performance in Africa. In his free time, he enjoys reading novels, playing football and watching documentaries about artificial intelligence and its application in healthcare.
Project Summary
Project Title: Monitoring Routine Health Indicators from District Health Information System (DHIS2): A Statistical Subsampling Approach
Abstract
Background: In Kenya, routine data is collected using the District Health Information System (DHIS2). This data is continuously collected and cheaper to obtain compared to surveys. Currently, there has been increased advocacy for using this data by governments and development organizations such as the World Health Organization (WHO) but it is unclear about how much DHIS2 data one needs to estimate indicators. All the studies that have used routine data use all the available reports to obtain estimates. This study proposes a novel sub-sampling approach to the estimation of indicators from routine data. This study hypothesized that subsamples of routine data can still provide credible estimates. Methods: We used data from 1,808 health facilities in Western Kenya from DHIS2. Information of 5 data elements, we computed three indicators, namely; the coverage of the third dose of pentavalent vaccine (DPT3), the proportion of pregnant women who receive LLINs, and the proportion of pregnant women who completed at least 4 ANC visits. The study then uses both spatial and non-spatial sampling to obtain proportions of data (90%, 80%, 70%, 60%, 50%, 40%, 30%, and 20%) from the entire dataset and compute estimates with corresponding confidence intervals (CIs). A z-test and power calculations were done to test for significant difference between the subsample estimates and the population estimates. Results: There was no significant difference between the population estimate and sub-sample estimates (all p-values > 0.05). However, smaller samples exhibited large CIs, mostly below 60% sample size. Conclusion: The results of this study imply that one doesn't need a universe of all health facilities to obtain estimates from DHIS2. The power calculation also supported this conclusion. However, based on the CIs of the estimates, we recommend sample sizes above 60%. Keywords: Routine data, Health facility data, Monitoring Indicators, Spatial sampling